167 - AI Product Management and Design: How Natalia Andreyeva and Team at Infor Nexus Create B2B Data Products that Customers Value
Experiencing Data w/ Brian T. O’Neill (UX for AI Data Products, SAAS Analytics, Data Product Management) - A podcast by Brian T. O’Neill from Designing for Analytics - Tuesdays
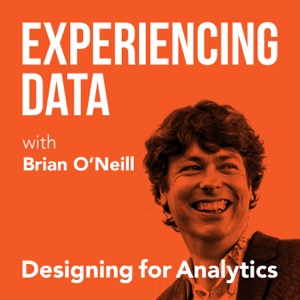
Categories:
Today, I’m talking with Natalia Andreyeva from Infor about AI / ML product management and its application to supply chain software. Natalia is a Senior Director of Product Management for the Nexus AI / ML Solution Portfolio, and she walks us through what is new, and what is not, about designing AI capabilities in B2B software. We also got into why user experience is so critical in data-driven products, and the role of design in ensuring AI produces value. During our chat, Natalia hit on the importance of really nailing down customer needs through solid discovery and the role of product leaders in this non-technical work. We also tackled some of the trickier aspects of designing for GenAI, digital assistants, the need to keep efforts strongly grounded in value creation for customers, and how even the best ML-based predictive analytics need to consider UX and the amount of evidence that customers need to believe the recommendations. During this episode, Natalia emphasizes a huge key to her work’s success: keeping customers and users in the loop throughout the product development lifecycle. Highlights/ Skip to What Natalia does as a Senior Director of Product Management for Infor Nexus (1:13) Who are the people using Infor Nexus Products and what do they accomplish when using them (2:51) Breaking down who makes up Natalia's team (4:05) What role does AI play in Natalia's work? (5:32) How do designers work with Natalia's team? (7:17) The problem that had Natalia rethink the discovery process when working with AI and machine learning applications (10:28) Why Natalia isn’t worried about competitors catching up to her team's design work (14:24) How Natalia works with Infor Nexus customers to help them understand the solutions her team is building (23:07) The biggest challenges Natalia faces with building GenAI and machine learning products (27:25) Natalia’s four steps to success in building AI products and capabilities (34:53) Where you can find more from Natalia (36:49) Quotes from Today’s Episode “I always launch discovery with customers, in the presence of the UX specialist [our designer]. We do the interviews together, and [regardless of who is facilitating] the goal is to understand the pain points of our customers by listening to how they do their jobs today. We do a series of these interviews and we distill them into the customer needs; the problems we need to really address for the customers. And then we start thinking about how to [address these needs]. Data products are a particular challenge because it’s not always that you can easily create a UX that would allow users to realize the value they’re searching for from the solution. And even if we can deliver it, consuming that is typically a challenge, too. So, this is where [design becomes really important]. [...] What I found through the years of experience is that it’s very difficult to explain to people around you what it is that you’re building when you’re dealing with a data-driven product. Is it a dashboard? Is it a workboard? They understand the word data, but that’s not what we are creating. We are creating the actual experience for the outcome that data will deliver to them indirectly, right? So, that’s typically how we work.” - Natalia Andreyeva (7:47) “[When doing discovery for products without AI], we already have ideas for what we want to get out. We know that there is a space in the market for those solutions to come to life. We just have to understand where. For AI-driven products, it’s not only about [the user’s] understanding of the problem or the design, it is also about understanding if the data exists and if it’s feasible to build the solution to address [the user’s] problem. [Data] feasibility is an extremely important piece because it will drive the UX as well.” - Natalia Andreyeva (10:50) “When [the team] discussed the problem, it sounded like a simple calculation that needed to be created [for users]. In reality, it was an entire p